Rajiv Chandrasekaran
In
The Paradox of Marketing Personalization
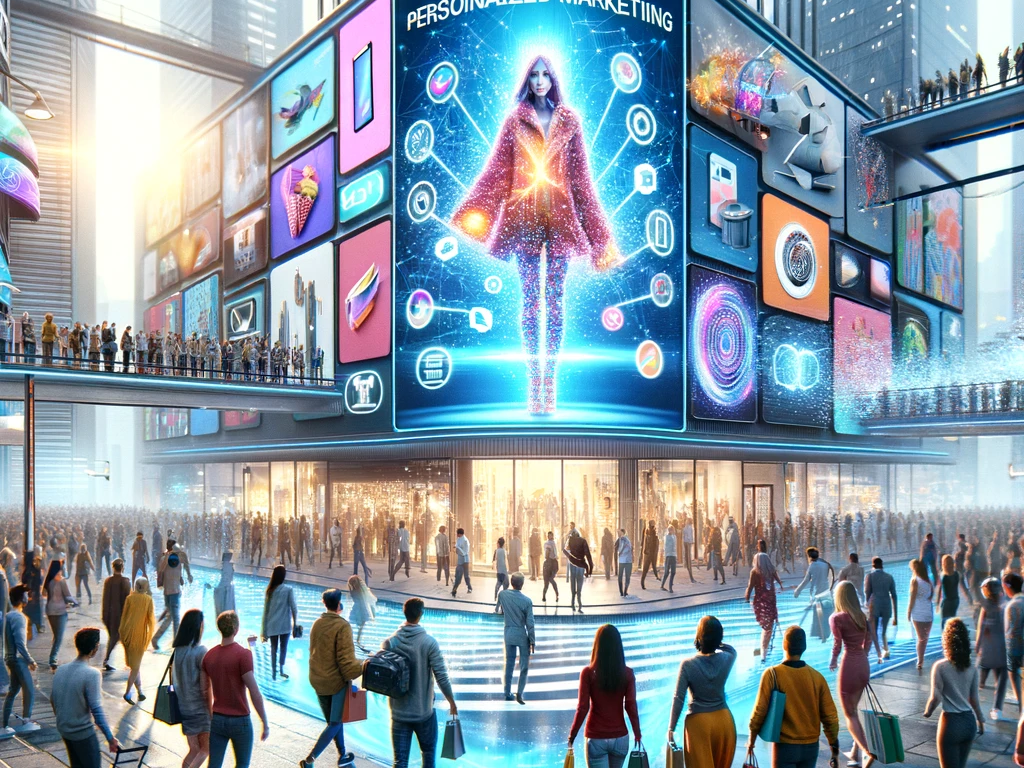
Marketing Personalization
Aggregating Data for Success While Avoiding the False Consensus Effect
Highlights
- Marketing personalization is based on the aggregation of data from multiple customers and products.
- Customers are not unique, which is why personalization works.
- Conversely, marketers must recognize that individual customer behaviors exhibit enough variation, so that projecting a specific customer’s traits onto a cohort (false consensus effect) may lead to incorrect conclusions.
Open up the Netflix portal, and you are immediately presented with an array of curated shows and movies, seemingly custom-tailored to your tastes. Open up the Panera App, and you immediately see an offer for a sandwich or baked good that looks mighty appealing to you. This is marketing personalization in action. Machine learning algorithms are constantly observing, tracking, and learning from your behavior to present you with offers that the algorithms believe you will accept. It is easy to feel that you, the customer, are indeed unique, and that companies have found a way to reach out to the real you. In fact, the whole process is presented as 1-to-1 marketing, with every customer being served a product, service, or solution uniquely customized to their needs.
How much of this is true? Are you, the customer, justified in thinking that you are indeed unique? And are there logical fallacies in your decision-making in believing so? How about the marketer? Are they making the correct decisions based on this framework? I will attempt to explore some of these questions in this post.
Myth #1: I, the customer, am special. I am not like the others
Let's briefly understand how these algorithms work. I will use the Pandora music engine as an example, but the concepts hold broadly. These algorithms work along two dimensions - namely, the product and customer dimensions. Products and customers are both characterized using multiple features. For example, Pandora classifies songs using "genes," analogous to the genes for living organisms, where these genes may correspond to musical traits such as tone, rhythm, type of instrument, meter, genre, and gender of the vocalist. Likewise, traits such as age, gender, geographical location, medium of usage, and time of day are used to characterize customers or their behaviors. When the machine learning is making the user a recommendation, it uses one of three methods:
i) it recommends songs (products) that are similar genetically to other songs that the user has heard before, or
ii) it recommends products (songs) bought (listened to) by other customers similar to the user, or
iii) uses a combination of the two approaches.
More advanced algorithms, such as collaborative filtering, use the same principles, except that the features ("genes") of the products and/or customers may be machine-generated, rather than human-curated. Now, back to the central question - if these recommendations are indeed personalized, why can't we think of them as a unique insight into a specific user's tastes? The simple reason is that despite the classification of songs (product) and customers using multi-dimensional features, the algorithm still relies on aggregating the results from multiple products and customers that are similar to a given target user.
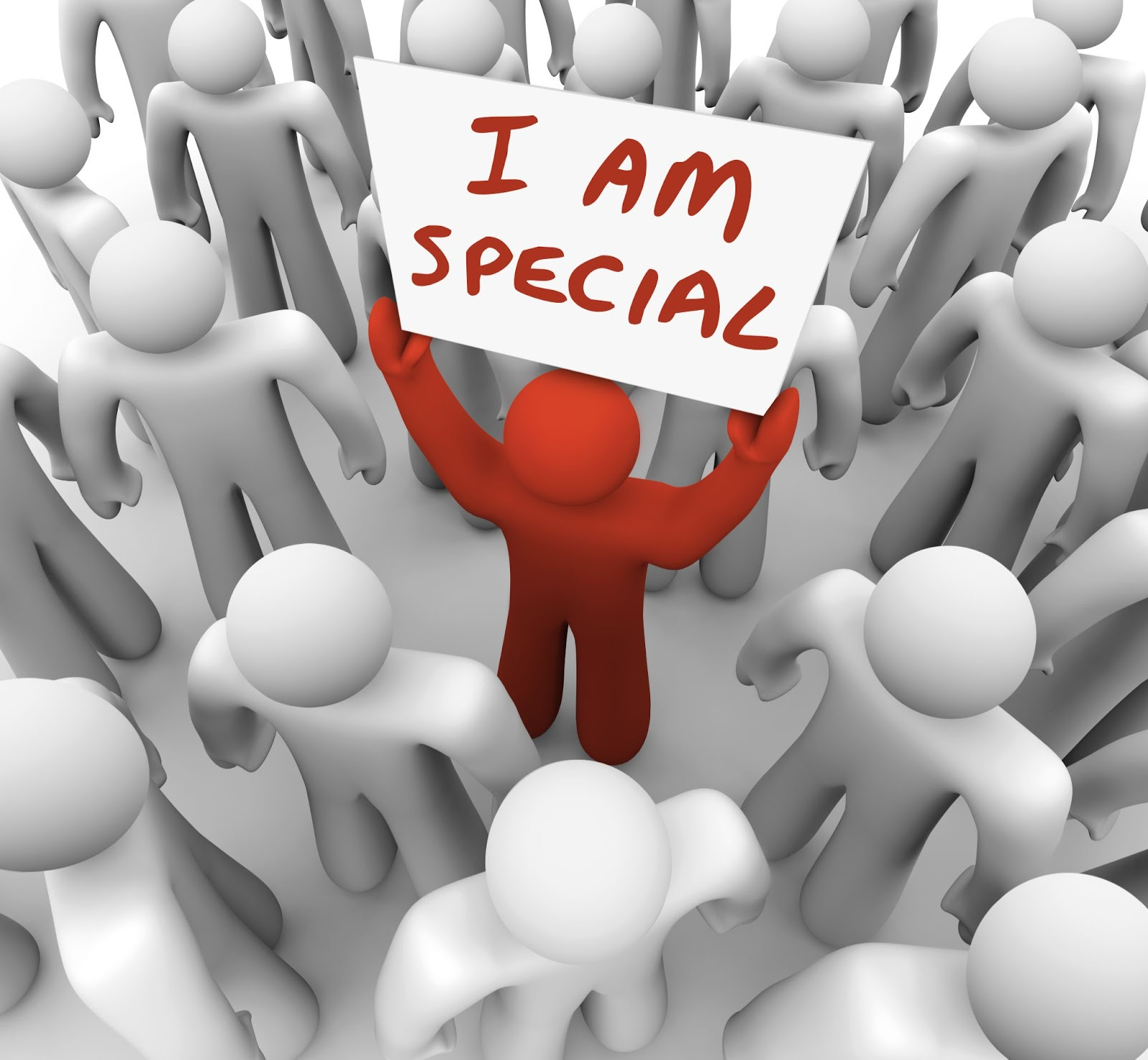
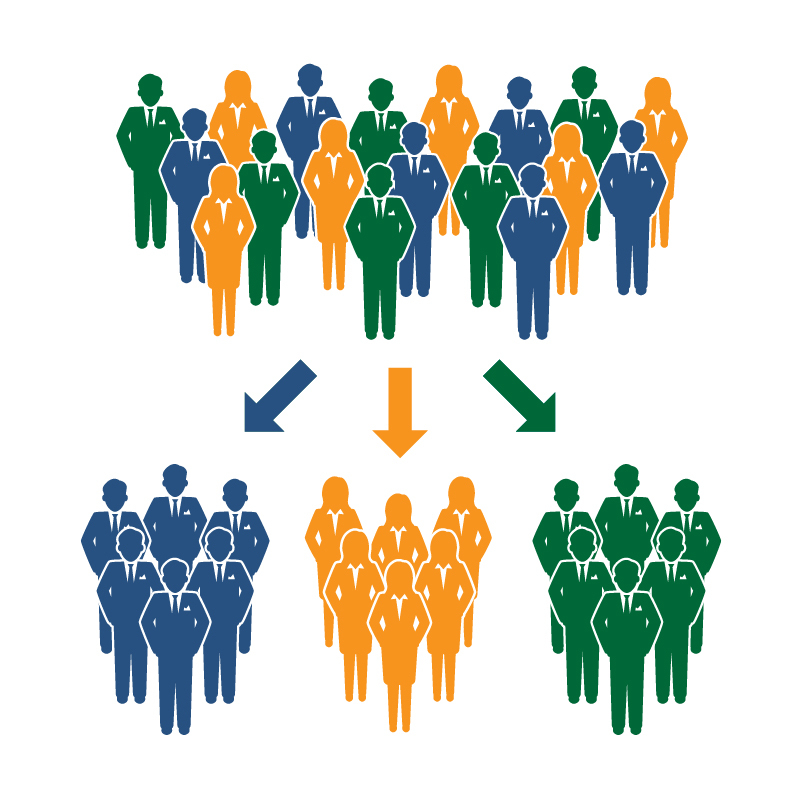
That is, there are indeed many more customers very similar to any given user, and that is the basis for the recommendation. In summary, customers are not unique. If a customer was genuinely unique in that there is no other customer with similar traits, the algorithm would be hard-pressed to present such a user with a good recommendation; in such cases, the recommendation would be no better than a random guess. To summarize, personalization works precisely because customers and products are not unique in every feature.
Myth #2: Others customers are like me
This myth seemingly contradicts Myth #1, but it reflects an error many marketers may make while designing marketing personalization algorithms. The error, known as the False Consensus Effect, is a result of marketers imagining themselves in the shoes of a potential customer, and consequently going on to believe that their personal choices are reflective of that of a large cohort of customers. How does this happen?
Quantifying Loyalty - A Quick Sidebar
There are two ways to measure loyalty: high-frequency loyalty (customers who buy your product more often) and sole-loyalty (customers who buy your product exclusively).
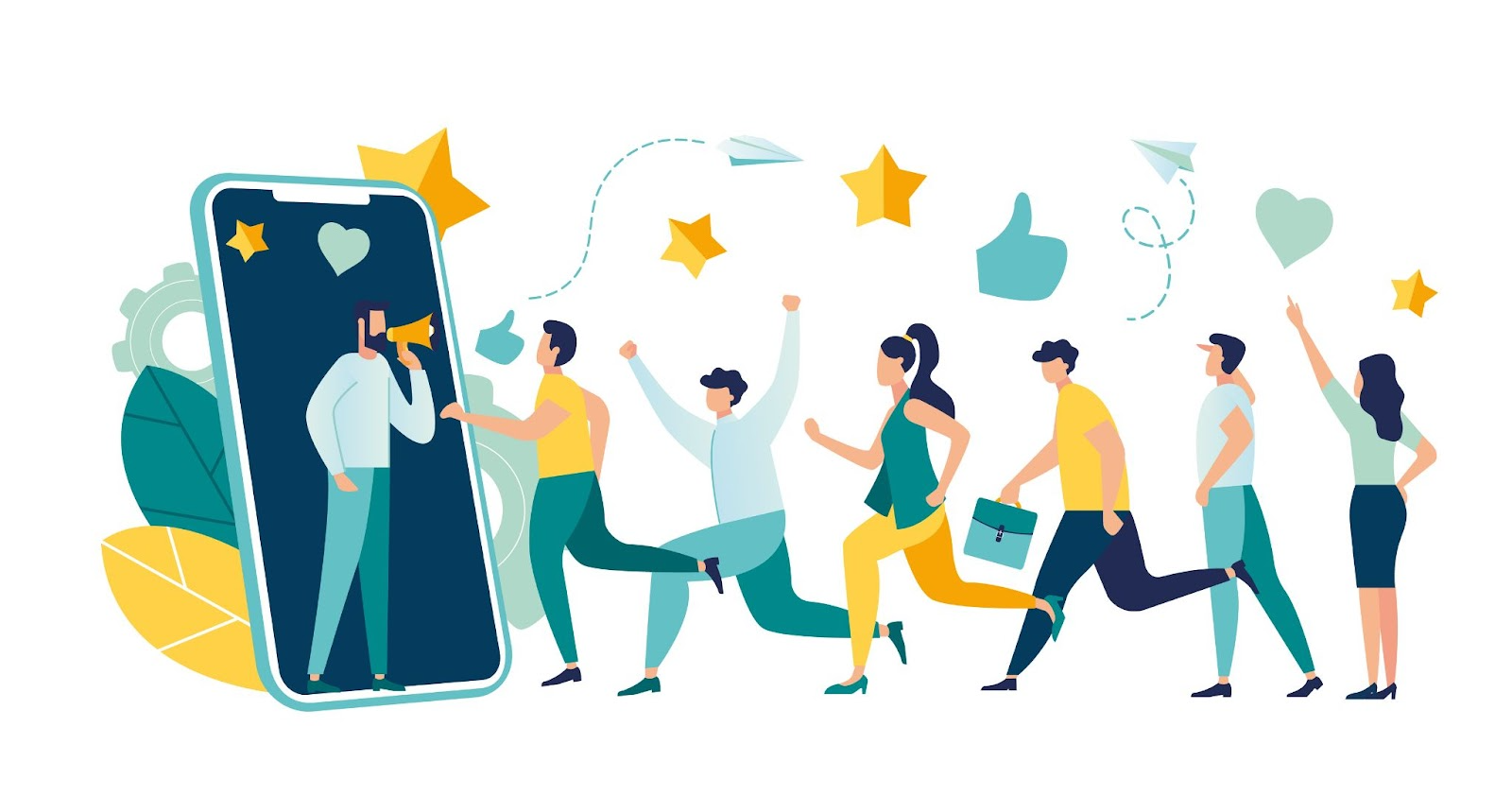
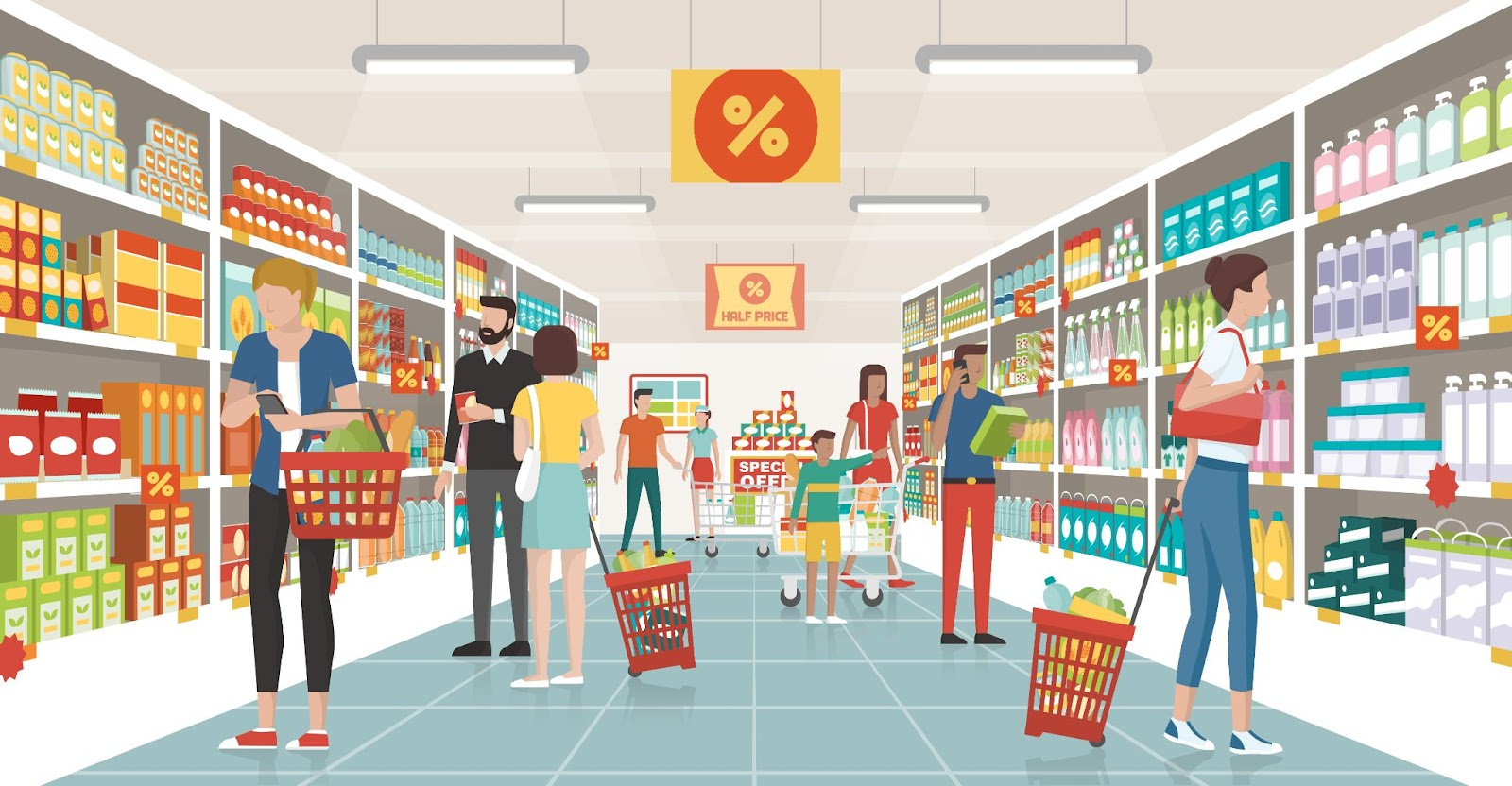
Empirical laws like the Double Jeopardy law in Marketing show that these two dimensions of loyalty do not move together, and that, in fact, they are negatively correlated. That is, high-frequency customers (heavy users of a particular product or service), may purchase from multiple brands within a specific category. Consequently, high-frequency customers are more likely to switch brands and tend to be less solely-loyal than low-frequency customers. In other words, solely-loyal customers are usually low-frequency customers.
This phenomenon is observed because if brands sell products that are broadly interchangeable, a statistical selection effect occurs, resulting in customers switching their preferences to brands that are more available mentally, physically, and economically. In other words, brands with broader customer reach (and therefore larger market shares) are more easily substitutable by other similar brands.
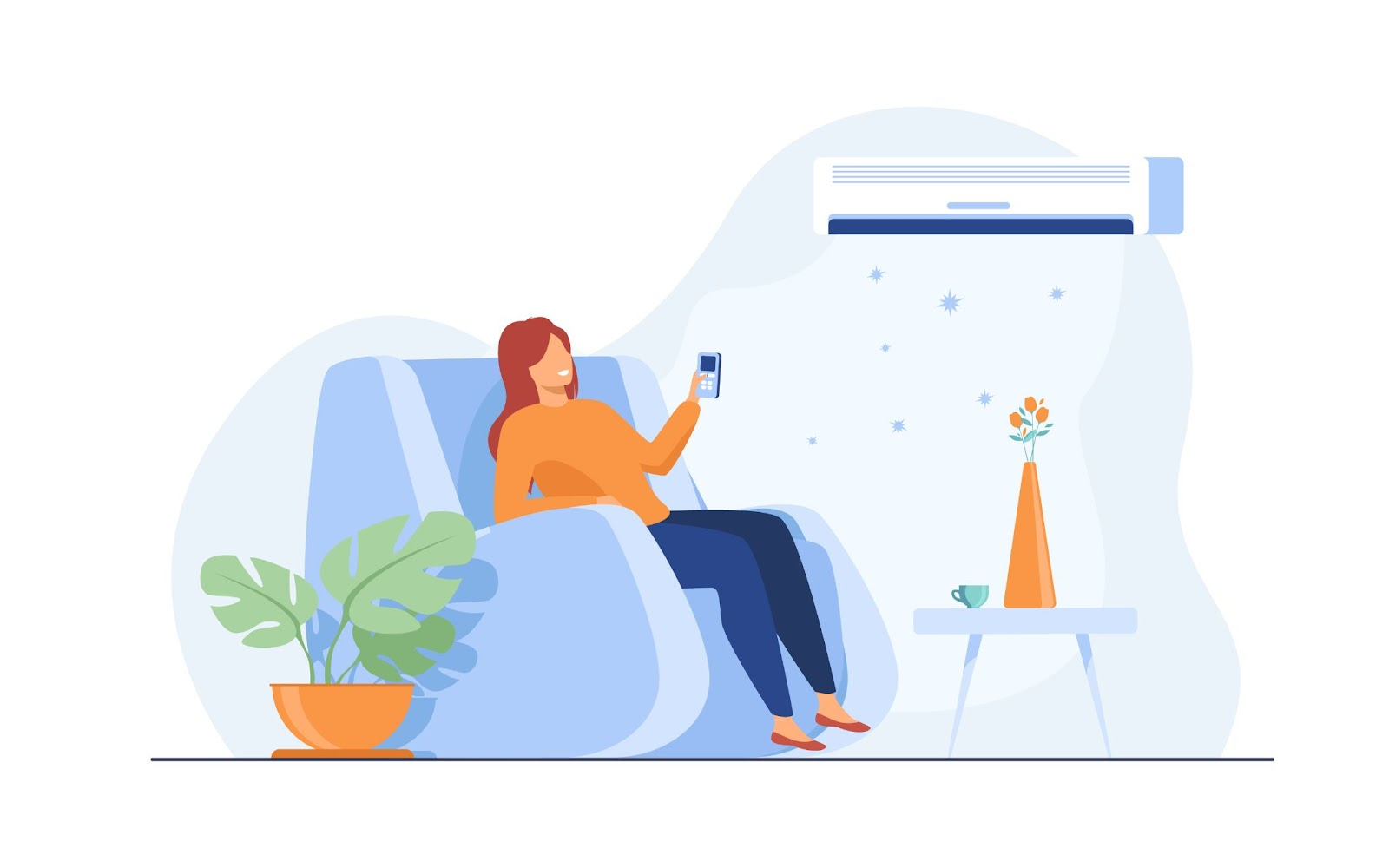
Let’s now look at a quick example to see how Myth #2 manifests from the perspective of a manager of a market-leading brand in a major category. The marketer imagines herself as a customer of a brand with large market share and concludes that she would never switch brands. And consequently, she extrapolates to reason that other customers like her are unlikely to switch too. That is, because she perceives herself as being solely loyal to the brand, she concludes that other customers must be like her as well. This is the False Consensus Effect in play in believing that others are like you. When, in fact, empirical evidence shows that other customers are not only likely to switch brands, but are more likely to do so if they are loyal (high-frequency customers).
In Conclusion
Marketers must recognize that in brands with large market shares, customer behaviors can only be understood in aggregate. Individual customer behaviors exhibit enough variation that projecting a specific customer trait into a cohort (“others are like me”) may lead to incorrect conclusions. Conversely, cohorts can only be actioned or acted upon in aggregate because individual customer behaviors are not predictable.
A useful analogy is to imagine the customers as individual molecules in a gas jostling about and bumping into each other vying for attention. No molecule is like any other, and attempting to predict the motions of individual molecules (customers) is futile. However, the aggregate behavior of these molecules (think temperature/humidity/pressure or reach/frequency/share-of-voice) can be well understood and acted upon and controlled using local actions. The air conditioner in a hot sweltering room can work well, though we cannot predict the motions of the individual molecules.
All bLogs